Mon, 23 Sep 2024 9:00 AM - 2:00 PM
C2D3 seeks to create an interdisciplinary data science and AI community for Early Career Researchers (ECRs) and students, as a place for supporting researchers and their ideas, sharing solutions and networking.
This half-day conference will provide a forum to exchange ideas, discuss research problems and solutions, and make new connections. During the conference we will hear presentations from the C2D3 ECR Seed Fund Awardees (2023 and 2024) and lightning talks from the ECR and student community.
Programme
09.00-09.20 Registration
09.20 -09.30 Opening with Matt Castle (Cambridge Centre for Data-Driven Discovery / Genetics)
Session 1 : Showcase ECR Seed Fund 2023 Awardee talks Chair: Mohammadreza Noormandipour (Physics)
- Simon Carringnon Beyond Projections: An Interdisciplinary Investigation of Socio-Economic Processes in Sustainable Food System Adoption using Agent Based Models of Cultural Evolution (McDonald Institute for Archaeological Research)
- Liz Yuanxi Lee AI-guided marker for early dementia predication (Adaptive Brain Lab, Psychology)
- Mia Tackney Using Digital Technologies in Clinical Trials for Pulmonary Hypertension (MRC Biostatistics Unit)
Q&A
Session 2 : Showcase: ECR Seed Fund 2024 Awardee talks Chair: Xin Du (Physics)
- Katherine Brown Automated detection and annotation of RNA virus subgenomic RNAs through analysis of public RNA-seq data (Pathology)
- Julian Gilbey Improving validation data strategies for machine learning in medicine (Applied Maths and Theoretical Physics)
- Dami Collier AI Advancements in HIVAN: Predicting Progression to ESRD (Pathology)
Q&A
- Ryan Daniels / Catherine Breslin Introducing AI Clinic (Accelerate Programme for Scientific Discovery)
11.05-11.35 Tea/Coffee Break
Session 3 : Lightning Talks "I have a solution to share" Chair: Jun Jie Peng (Cancer Research UK)
- Jack Atkinson FTorch: A library for coupling PyTorch machine learning models into large Fortran codes (Institute of Computing for Climate Science)
- Tristan Whitmarsh A new software tool for the visualization, annotation, and segmentation of biomedical images (Astronomy)
- Shrankhla Pandey Automatic annotation of mental health recovery narratives using NLP methods (Computer Science and Technology, Psychiatry)
- Mohammadreza Noormandipour Solving Drug Discovery Optimization Problems with Ising Machines (DAMTP, Physics)
"I have a problem to solve"
- Zakhar Shumaylov AI Models Collapse When Trained on Recursively Generated Data (Applied Maths and Theoretical Physics)
- Guillaume Proffit Historical Census Linking: issues of scale (History)
- Lloyd Fung Fast Bayesian Model Identification for Control (Applied Maths and Theoretical Physics))
Q&A: Problem solving discussions
12.55-14.00 Light lunch & networking
Lightning Talk Abstracts - Confirmed
Jack Atkinson FTorch: A library for coupling PyTorch machine learning models into large Fortran codes (Institute of Computing for Climate Science)
Abstract: Many large modelling codes are seeking to incorporate recent developments in machine learning (ML). This is often done through ML working in conjunction with the existing numerical codes rather than as a complete replacement. Doing so introduces a number of challenges around reproducibility, re-usability, and language interoperation - ML development is usually conducted in Python whilst numerical scientific codes are often written in compiled languages like Fortran, C, or C++. These challenges form a barrier to researchers increasing complexity and ‘time-to-science’. We present FTorch, a library developed in the Institute of Computing for Climate Science (ICCS) at the University of Cambridge. The aim of this is to make it easy for researchers to develop ML models in the PyTorch framework and couple them to large numerical codebases in Fortran. Particularly in mind are those without an extensive computing background or access to software/computing support. Whilst its origins lie in the domain of weather and climate research we have since had interest from users in molecular modelling, astrophysics, civil engineering, and other scientific domains. https://github.com/Cambridge-ICCS/FTorch
Lloyd Fung Fast Bayesian Model Identification for Control (Applied Maths and Theoretical Physics)
Abstract: We pose the following problem in control theory: Assuming a control device has access to and control over the full state space of a dynamical system but does not have any prior knowledge of the governing equation of a dynamical system, what is the quickest and most optimal way for the control system to explore the state space and learn about the equation governing the dynamical system while controlling its desired state?
This is a generic problem for a lot of control engineering and may have real-world potential in applications such as drones and aircraft control when abrupt changes in the system require the controlling device to learn about new dynamics in real-time. It is also effectively a reinforcement learning problem, as the control device is required to learn about the system while balancing exploration and exploitation. However, different from many trendy reinforcement learning techniques, we constrain ourselves to models that can be written as dyanmical equations. To this end, the Sparse Identification of Nonlinear Dynamics (SINDy) framework has been shown to be effective in learning interpretable and parsimonious equations directly from data. However, it is not the most robust against noise and requires a lot of data to learn models properly. Our current work recasts SINDy in a Bayesian framework. By taking into account the uncertainty around the data, our method shows a more robust capability for learning the correct model in the low-data limit. Moreover, our method approximates the Bayesian likelihood and evidence, avoiding the need for computationally expensive Markov chain Monte Carlo (MCMC) sampling and enabling its use in real-time control applications.
By computing the potential information gain, the Bayesian approach can also inform optimal sampling and effectively form an active learning problem. The question remains, however, on how to incorporate this information into a control rule in optimal control theory.
Mohammadreza Noormandipour Solving Drug Discovery Optimization Problems with Ising Machines (Applied Maths and Theoretical Physics)
Abstract: In the rapidly evolving field of drug discovery, the ability to efficiently solve complex optimization problems is crucial for identifying novel therapeutic compounds, predicting drug-target interactions, and optimizing lead candidates. This talk will explore the application of Ising machines—powerful combinatorial optimization solvers—to key challenges in drug discovery. By mapping problems such as protein-ligand docking, molecular conformation optimization, and drug target interaction prediction onto the Ising model, we can leverage the unique capabilities of these machines to accelerate the discovery process. Specific use cases will include optimizing molecular conformations, designing new drug-like molecules, and predicting off-target effects, demonstrating how the combinatorial nature of these tasks can be effectively addressed using Ising-based approaches. Attendees will gain insight into the practical implementation of Ising machines and the potential for these tools to revolutionize the optimization landscape in pharmaceutical research.
Shrankhla Pandey A new software tool for the visualization, annotation, and segmentation of biomedical images (Astronomy)
Abstract: Mental health recovery narratives can provide recovery-oriented benefits [1], including increased quality of life and the presence of meaning in life. Our objective is to develop an automated system that can categorize recovery narratives with respect to seven dimensions, including genre, tone, and trajectory. We will achieve this by utilizing both natural language processing (NLP) and acoustic speech features, employing machine learning techniques.
[1] Slade et al. Recorded Mental Health Recovery Narratives as a resource for People Affected by Mental Health Problems.
Guillaume Proffit Historical Census Linking: issues of scale (History)
Abstract: Individual-level historical census for the decades 1851 to 1921 have been made avaialable to population researchers, and already been transformative. However, there is immense value in linking individuals across these censuses to follow evolution of individual or household chaarcteristics as causes or consequences of other factors (transports, demographic shifts, changes in the stucture of the economy, etc). Following litterature on American censuses, I have implemented a solution based on the Fellegi Sunter statistical model, but am facing issues with the scale of the computing: the worst case scenario to link two 30m censuses is to compare 9*10^12 pairs of records. These comparisons can be reduced thanks to blocking, but this risks creating false negatives. Even using other solutions such as an ML classifier, the core scale problem remains the same.
Zakhar Shumaylov AI Models Collapse When Trained on Recursively Generated Data (Applied Maths and Theoretical Physics)
Abstract: Stable Diffusion revolutionised image creation from descriptive text. GPT-2, GPT-3(.5) and GPT-4 demonstrated astonishing performance across a variety of language tasks. ChatGPT introduced such language models to the public. It is now clear that large language models (LLMs) are here to stay, and will drastically change the ecosystem of online text and images. In this talk we consider what may happen to GPT-{n} once LLMs contribute much of the text found online. We find that use of model-generated content in training causes irreversible defects in the resulting models, where tails of the original content distribution disappear. We refer to this effect as model collapse and show that it can occur in LLMs, as well as in Variational Autoencoders and Gaussian Mixture Models. We build theoretical intuition behind the phenomenon and portray its ubiquity amongst all learned generative models. We demonstrate that it must be taken seriously if we are to sustain the benefits of training from large-scale data scraped from the web. Indeed, the value of data collected about genuine human interactions with systems will be increasingly valuable in the presence of LLM-generated content in data crawled from the Internet.
Tristan Whitmarsh A new software tool for the visualization, annotation, and segmentation of biomedical images (Astronomy)
Abstract: ScanXm is a newly released software tool developed by Tristan Whitmarsh at the University of Cambridge. It aims to provide a simple and user-friendly interface for the annotation of 2D and 3D medical and biomedical images. ScanXm also includes several deep learning modules for image processing and the automatic segmentation of various organs and tissue types. Notably, this software requires no Python coding, will run without an expensive GPU, and does not use cloud computing, thus keeping your confidential patient data safe. Through a recent collaboration with NVIDIA, ScanXm now seamlessly integrates with MONAI Label. This integration enables ScanXm to run powerful AI models such as vision transformers. ScanXm now also links with Segment Anything 2 by Meta, which allows it to segment any object with only a few mouse clicks.
Organising Committee
Sireesha Chamarthi (Astronomy), Xin Du (Physics), Ed Harding (Clinical Biochemistry), Mohammadreza Noormandipour (Physics), Parley Ruogu Yang (Applied Maths and Theoretical Physics), Jun Jie Peng (Cancer Research UK), Anna Maria Papameletiou (Cancer Research UK), Maha Waseem (Judge Business School), Qiyao Wei (Applied Maths and Theoretical Physics), C.Zhang (Obstetrics and Gynaecology).
Registrations
Closed.
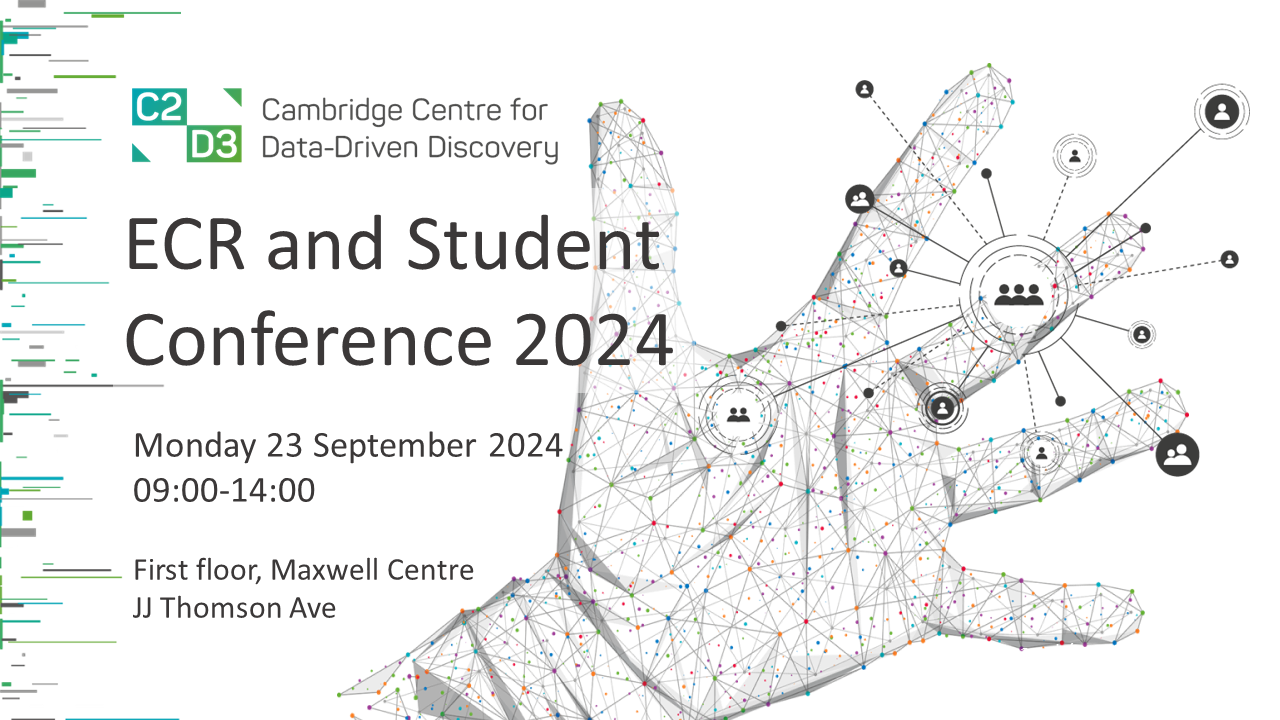
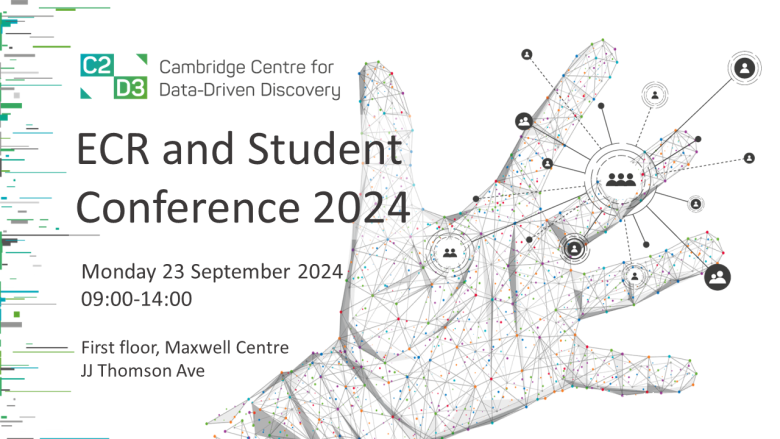