Trustworthy AI for Medical and Health Research Workshop
Artificial Intelligence (AI) systems are increasingly being deployed in society, it is critical to ensure that these systems are trustworthy, in particular in high-stake real-world applications, such as the medical and health domains. There has been growing interest to develop and deploy AI models and algorithms that are not only accurate, but also interpretable/explainable, fair, privacy-preserving, causal, and robust. This Trustworthy Artificial Intelligence for Medical and Health Research workshop will focus on frontier research in this timely, trending, and important cross-disciplinary research domain. It will provide an excellent opportunity to establish and expand the academic networks of involved researchers, as well as to share cutting-edge knowledge, technology, and findings. The workshop will also involve a follow-up seminar series to strengthen and deepen the established networks to inspire new ideas and foster academic collaborations.
Follow-on Event - Monday 23 January 2023, 11:00-12:00
Speaker: Matthew Juniper, Professor of Department of Engineering in University of Cambridge.
Audience
This workshop is for PhD students, postdocs or other early career researchers.
Short talk or poster presentation opportunities
There may be opportunities to give a short talk or present a poster on relevant subjects. Please email Dr Pingfan Song to discuss the options available.
Registration
Registration is now closed.
Programme
13:30 - 13:50 Arrival and registration
13:50 - 14:15 Welcome and Opening remarks
Introduction to C2D3 (Dr Pingfan Song)
Themed speech from the organisers (Dr Pingfan Song)
Session 1: Chair - Dr Lefan Wang (Engineering)
14:15 - 15:00 Keynote Speaker: Dr Li Su (Psychiatry)
15:00 - 15:30 Short Talks: Prof Matthew Juniper (Engineering); Dr Michael Burkhart (Psychology)
15:30 - 16:00 Refreshment Break and Poster session
Session 2: Chair - Dr Vihari Piratla (Engineering)
16:00 - 17:00 Keynote Speaker: Dr Alexander Gimson (Cambridge Centre for Artificial Intelligence in Medicine)
17:00 - 17:30 Short Talks: Dr Yuanxi Li (Psychology); Dr Michael Roberts (DAMTP)
17:30 - 18:00 Networking and drinks reception
Speaker and Chairs Abstracts and Biographies
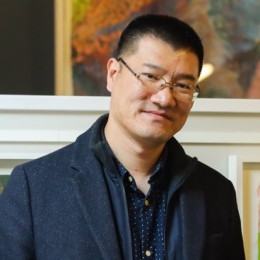
Professor Li Su
Abstract:
Integrating AI and neuroimaging with neurobiologically plausible cognitive models
Biography:
Professor Li Su is the head of the Artificial Intelligence and Computational Neuroscience Group and has a joint appointment as Principal Research Fellow in the Department of Psychiatry at the University of Cambridge and Professor of Neuroimaging in the Department of Neuroscience in University of Sheffield. His research aims to combine innovative and original computational methods with the state-of-the-art brain imaging techniques (MEG/EEG, 7T MRI and PET) in understanding, detecting and developing treatments for neurological and psychiatric conditions. He has been awarded a prestigious Senior Fellowship from Alzheimer’s Research UK in 2017 and fellow of Clare Hall in 2018.

Professor Matthew Juniper
Abstract:
From wings to hearts: physics-based machine learning for flow-MRI
Flow-MRI is a non-invasive imaging method that generates 3-component velocity fields of opaque flows, such as blood, in three spatial and one time dimension. It is becoming clinically established on state-of-the-art MRI systems. Achieving acceptable signal-to-noise ratio (SNR) and velocity-to-noise ratio (VNR), however, requires long acquisition times and coarse spatial resolutions. These limitations mean that it cannot compete with the accuracy of invasive cardiac catheterisation or the convenience of transthoracic echocardiography.
Our solution is to use one crucial piece of information that has so far been ignored: that the image is of blood flow through a tube. We know that the flow obeys the Navier–Stokes equations and that, at these scales, the fluid is Newtonian. We can therefore take a single flow-MRI image, even if it is severely corrupted by noise, and calculate the flow and the boundaries that are most likely to have given that image. This uses Bayesian inference, shape optimization, and adjoint-enabled CFD, which were originally pioneered for aerospace applications. Highly accurate derived information such as the pressure field, kinetic and viscous energy losses, and vessel wall shear stress follow at no extra cost. Instead of using Neural Networks (NNs), we assimilate the data directly into a Navier-Stokes boundary value problem. The physics is therefore hard-wired into the model, rather than applied as a soft constraint on a NN. This avoids many of the trustworthy problems that arise from using NNs.
Biography:
Matthew Juniper is Professor of Thermofluid Mechanics at the Engineering Department and is the Principal Investigator of the UK Fluids Network (www.fluids.ac.uk). He completed his PhD from Ecole Central Paris in 2001 and was appointed Lecturer in Combustion at the University of Cambridge in 2003. His research interests are in the broad area of flow instability, adjoint methods, shape optimization, and physics-based Bayesian inference. He is an Associate Editor of the Journal of Fluid Mechanics and has held visiting fellowships/professorships at Ecole Central Lyon, the Institute for Advanced Studies at TU Munich, KTH/Nordita Stokholm, IIT Madras, and the Center for Turbulence Research Summer Program at Stanford University.
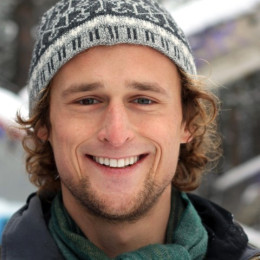
Dr Michael Burkhart
Abstract:
Clustering trajectories of neurodegenerative disease with mixtures of state space models
We propose and validate a mixture of state space models to perform unsupervised clustering of short trajectories. Within the state space framework, we let expensive-to-gather biomarkers correspond to hidden states and readily obtainable cognitive metrics correspond to measurements. Upon training with EM, we find that our clusters tend to stratify persons according to clinical outcome. Furthermore, we can effectively predict on held-out trajectories using cognitive metrics alone. Our approach accommodates missing data through model marginalisation and generalises across research and clinical populations.
Biography:
Michael Burkhart earned his Ph.D. in 2019 from Brown University's Division of Applied Mathematics. He and his advisor, Matthew Harrison, collaborated with the BrainGate clinical trial that develops intracortical brain–computer interfaces to enable persons with quadriplegia to communicate and interact with their environments using mental imagery alone. Together with Leigh Hochberg and David Brandman, they derived and implemented a novel Bayesian filtering framework for neural decoding. In 2021, he joined the Adaptive Brain Lab at Cambridge University as a research associate to develop machine learning-based approaches for the early diagnosis of neurodegenerative disease. He's excited to continue working at the interface of artificial intelligence and neuroscience.
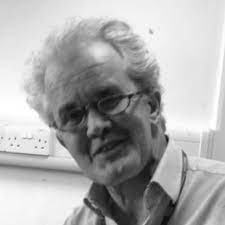
Dr Alexander Gimson
Abstract:
Developing trustworthy machine learning for organ transplantation
Biography:
Dr Alexander Gimson was a Consultant Transplant Hepatologist at Cambridge University Hospital NHS Foundation Trust, where he was previously Director of Medicine. He led the national team which developed new liver transplantation recipient selection criteria for the UK and introduced a novel national organ allocation offering scheme whereby organs are offered to the individual on a national waiting list who has the greatest calculated net life years gained from the particular donor organ. With professor van der Schaar, he has developed two new AI machine learning models which can improve on present Cox models with interpretable allocation policies. Developing clinically relevant and trustworthy ML models for both clinicians and patients within organ transplantation highlights a number of crucial areas that require more stringent examination.

Dr Yuanxi Li
Abstract:
Modelling prognostic trajectories of cognitive decline due to Alzheimer's disease
We propose a novel trajectory modelling approach based on metric learning (Generalised Metric Learning Vector Quantization) that mines multimodal data from MCI patients in the Alzheimer's disease Neuroimaging Initiative (ADNI) cohort to derive individualised prognostic scores of cognitive decline due to AD. We develop an integrated biomarker generationusing partial least squares regression– and classification methodology that extends beyond binary patient classification into discrete subgroups (i.e. stable vs. progressive MCI), determines individual profiles from baseline (i.e. cognitive or biological) data and predicts individual cognitive trajectories (i.e. change in memory scores from baseline).
Biography:
Yuanxi Li (Liz) gained a BEng and MEng in Electrical Engineering at Tsinghua University and a DPhil in Cognitive Psychology at Beijing Normal University. She joined Dr Zoe Kourtzi’s lab in 2022 as a Postdoctoral Research Associate, using machine learning-based approaches for the early diagnosis of neurodegenerative disease on the EDoN project. Her research interests focus on early detection of dementia, adaptive brain and healthy ageing intervention.

Dr Michael Roberts
Biography:
Dr Roberts is Senior Research Associate of Applied Mathematics at DAMTP and member of the Cambridge Image Analysis group (CIA) and leads the algorithm development team for the global COVID-19 AIX-COVNET collaboration, see https://covid19ai.maths.cam.ac.uk/.
Dr Roberts' research interests focus on variational methods for image processing (in particular image segmentation and registration), machine learning for image and data analysis, image processing and data analysis. He has active interdisciplinary collaborations with other applied mathematicians, computer scientists and clinicians focussing on medical imaging problems. He has vast experience in studying medical imaging problems for lung diseases including (but not limited to) lung cancer, idiopathic lung fibrosis, mesothelioma and drug induced interstitial lung disease.

Dr Lefan Wang
Biography:
Lefan Wang has joined the ifM as a Research Associate in the Complex Additive Materials Group. Before this, she worked as a postdoc researcher in the Mechanical Engineering at the University of Leeds. She received her PhD degree at the Cardiff University in 2018. Her research interests include advanced sensing techniques, mobile medical/healthcare systems, additive manufacturing, and data analysis based on machine learning.
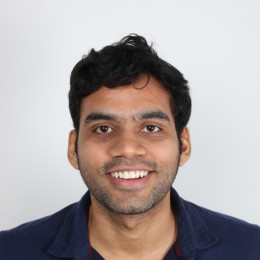
Dr Vihari Piratla
Biography:
Vihari Piratla a postdoc with the Machine Learning Group of Cambridge University, supervised by Dr Adrian Weller. From 2017-2022, he was a PhD student with the Computer Science department of IIT Bombay. He is passionate about research challenges that arise when deploying Machine Learning systems in the wild. He was awarded Google PhD Fellowship in 2020.
Posters:
Mr Cathal McCague, PhD student and radiology registrar (CRUK CI and Department of Radiology)
Poster title: Stop rolling the Dice
Authors: Cathal McCague, Thomas Buddenkotte, Ramona Woitek, Lorena Escudero Sanchez, David Hulse, Roxana Pintican, Pubudu Piyatissa, Syafiq Ramlee, Leonardo Rundo, Carola Bibane Schonleib, Ozan Oktem, Evis Sala, Mireia Crispin Ortuzar.
Affiliations: CRUK CI and Department of Radiology
Ms Elisa Gómez de Lope (University of Luxembourg)
Poster title: Machine learning applied to higher order functional representations of omics data reveals biological pathways associated with Parkinson Disease
Authors: Elisa Gómez de Lope1,2, Enrico Glaab1
Affiliations: 1 Biomedical Data Science Group, LCSB, University of Luxembourg; 2 Department of Computer Science and Technology, University of Cambridge (Visitor)
Organiser:
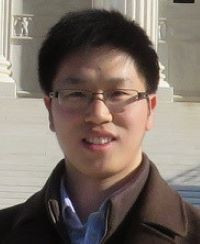
Dr Pingfan Song received his B.S. and M.S degree both from Harbin Institute of Technology (HIT), China, and his Ph.D. degree from University College London (UCL), UK. He was a research associate at Imperial College London, and is now a senior research associate at University of Cambridge. Pingfan has been working on trustworthy machine learning which aims to advance work on key technical underpinnings of interpretability/transparency, fairness, and robustness of machine learning systems in order to push forward the next-generation AI. In addition to machine learning, he is also a professional in image processing, sparse modeling and sampling theory. His research has been applied to multi-disciplinary fields such as medical imaging, biological imaging, and other computational imaging tasks and inverse problems.
Pingfan was one of the winners of the C2D3 Early Career Research Seed Fund 2022.
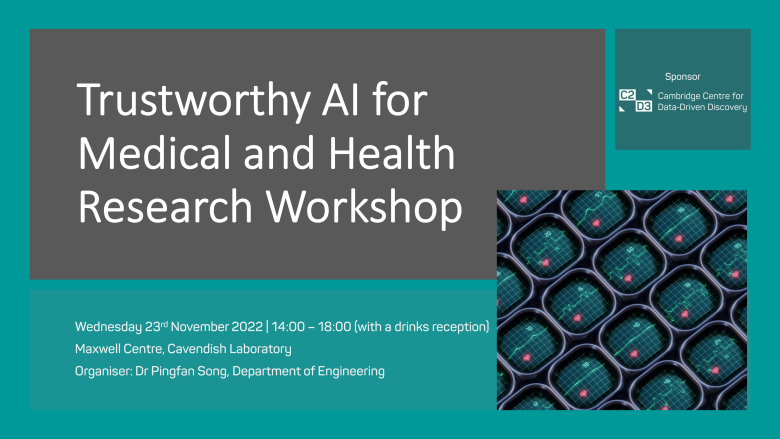